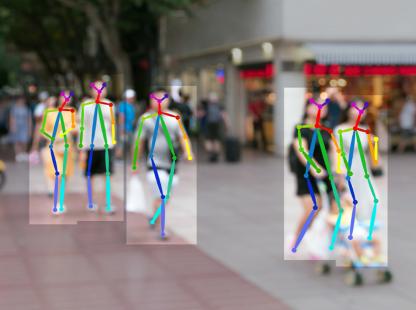
Image and Video
Explore our Research Topics
Chen Liu |
Research Areas: Computer vision, pattern and face recognition, video processing Making Intelligent Transportation Systems Smarter The New Jersey Department of Transportation designated more than 400 closed-circuit video cameras statewide for incident monitoring, traffic congestion control and public safety operations. Video streams from these cameras feed to a back-end system. There, video analytics software is used to perform target detection and incident monitoring applications. We are actively working on incorporating wireless sensor networks, hierarchical edge-computing and computer vision to mitigate the challenging problems in various illumination and weather conditions in order to achieve fast and automated video based traffic monitoring. Video Analytics Pilot Studies and Testing of Technologies We propose a new modular approach for statistical modeling of traffic incidents and model selection in order to improve state-of-the-art traffic detection and monitoring. We investigated and developed automated video analytics systems to replace human operators for traffic incident detection and to monitor the cameras installed along the major New Jersey highways. We test the proposed technologies and benchmark their performance. |
|
Frank Shih
|
Research Areas: Image processing, artificial intelligence, digital watermarking, digital forensics, robot sensing, neural networks Deep Learning for Pneumonia Classification and Segmentation on Medical Images Automatic identification of pneumonia on medical images has attracted intensive study. In this project, we develop a novel joint-task architecture that can learn pneumonia classification and segmentation simultaneously. Two modules, including an image preprocessing module and an attention module, are developed to improve both classification and segmentation accuracies. Experimental results performed on the massive dataset of the Radiology Society of North America have confirmed its superiority over other existing methods. Deep Morphological Neural Networks and Applications Given a target image, determining suitable morphological operations and structuring elements is a cumbersome and time-consuming task. In this project, we propose new morphological neural networks, which includes a nonlinear feature extraction layer to learn the structuring element correctly, and an adaptive layer to automatically select appropriate morphological operations. We also use them for their classification applications, including hand-written digits, geometric shapes, traffic signs and brain tumors. Experimental results show higher computational efficiency and higher accuracy when compared with existing convolutional neural network models.
|