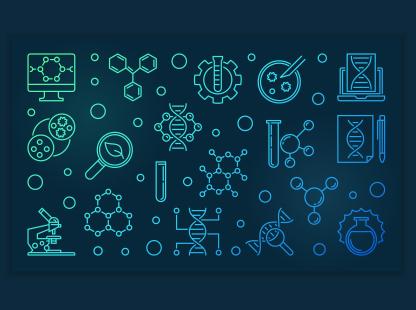
Bioinformatics and Medical Informatics
Our research is focused on advancing machine learning, statistical modeling, natural language processing and data analytics to tackle key challenges in biomedical fields. We work on developing novel deep learning methods for analyzing single-cell genomic data, improving medical informatics and creating medical ontologies. Recent projects include modeling Social and Commercial Determinants of Health, which significantly influence public health and building ontologies to better understand their impacts. Our work also applies deep learning to medical AI challenges, such as tumor identification in brain MRI images and vessel identification in vascular ultrasound and enhances electronic health records (EHR) usability through machine learning. This includes improving comprehension of clinical notes with large language models and other methods to simplify EHR text for patients. In addition, we are leveraging generative models to predict Alzheimer’s disease progression by addressing missing data issues and under-representation in marginalized populations. Our aim is to integrate complex, multimodal data for more accurate prediction models in aging-related diseases. Overall, our efforts focus on integrating biological information and computational techniques to improve both the analytical performance and interpretability of complex biomedical data.